Lorem ipsum dolor sit amet, consectetur adipiscing elit. Nullam consectetur, sapien a blandit sodales, leo libero lacinia metus, ac feugiat justo sapien et nunc. Curabitur pretium felis nec mi finibus, non fringilla nulla consequat. Donec facilisis, sapien id facilisis sagittis, dui libero semper lorem, id fermentum erat nunc vel sapien.
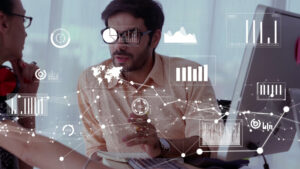